This week I start conducting experiments about long term tracking. Except for the experiments, I still read some papers about long term object tracking.
The experiments are as follows: On the basis of a simple siamese tracker(SiamFC++), I modified it to a long term tracker,which needs a mechanism to judge whether the target object is absent. The most concise way is to exploit the confidence score. So I want to find a relationship between the score and IOU. The specific results are shown in the figure.

From the picture, we can see that the fluctuation trend of score is basically consistent with that of iou. But only relying on the score is not enough. So we may conduct experiments on the sequence of scores.
The new papers are as follows:
I change my mind that I should read more new papers instead of conducting experiments in haste. The specific analysis of papers are as follows:
1. Adaptive and Background-Aware Vision Transformer for Real-Time UAV Tracking
The paper’s purpose:
To slove the problem that traditional CNN is too slow.
Contributions:
(1) The paper proposes a framework, where feature learning and template-search coupling are integrated into an efficient one-stream ViT to avoid an extra heavy relation modeling module.
(2)The proposed Aba-ViT exploits an adaptive and background-aware token computation method to reduce inference time.
(3)This approach adaptively discards tokens based on learned halting probabilities, which a priori are higher for background tokens than target ones.
(4) Very Fast ! 180 fps!
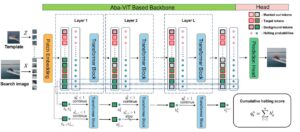
Personal Evaluation:
Too fast! And the paper provide the code. We can let it act as main tracker.
2. Boosting UAV Tracking With Voxel-Based Trajectory-Aware Pre-Training
The paper’s purpose:
(1)To slove the problem that the siamese tracker was trapped when facing multiple views of object in consecutive frames.
(2)The general image-level pretrained backbone can overfit to holistic representations, causing the misalignment to learn object-level properties in UAV tracking.
Contributions:
(1) Fully exploit the stereoscopic representation for UAV tracking. Specifically, a novel pre-training paradigm method is proposed.
(2) Through trajectory-aware reconstruction training (TRT), the capability of the backbone to extract stereoscopic structure feature is strengthened without any parameter increment.
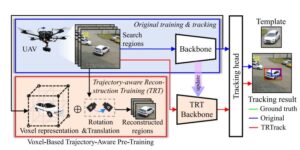
Personal Evaluation:
No code. The paper is related to 3D tracking.
3. Compact Transformer Tracker with Correlative Masked Modeling
The paper’s purpose:
(1) Proving that the traditional selfattention structure is sufficient for information aggregation, and structural adaption is unnecessary.
Contributions:
(1) The paper attaches a lightweight correlative masked decoder which reconstructs the original template and search image from the corresponding masked tokens.
(2) The structure is very simple.
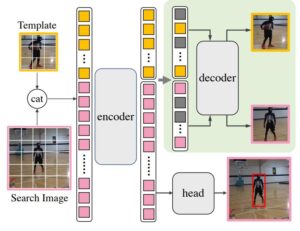
Personal Evaluation:
Release code. The evaluation on benchmark is very high. Nice paper. But the analysis of network is beyond my ability.
4. Continuity-Aware Latent Interframe Information Mining for Reliable UAV Tracking
The paper’s purpose:
(1) Mainly focuses on explicit information to improve tracking performance, ignoring potential interframe connections.
Contributions:
(1) A network can generate highly-effective latent frame between two adjacent frames.
(2) Fully explore continuity-aware spatial-temporal information.

Personal Evaluation:
Release code. The innovation points are very innovative.